Conditional Entropy¶
The conditional entropy period finder [G2013] phase-folds the data at each trial frequencies and estimates the conditional entropy \(H(m|\phi)\) of the data. The idea is that the data with the least entropy (intuitively: the greatest “structure” or “non-randomness”), should correspond to the correct frequency of a stationary signal.
Here,
where \(p(m, \phi)\) is the density of points that fall within the bin located at phase \(\phi\) and magnitude \(m\) and \(p(\phi) = \sum_m p(m, \phi)\) is the density of points that fall within the phi range.
(Source code, png, hires.png, pdf)
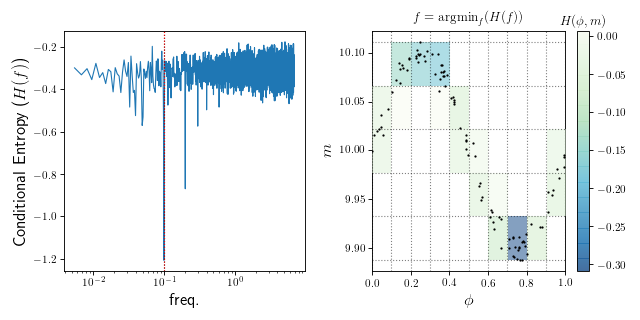
An example with cuvarbase
¶
import cuvarbase.ce as ce
import numpy as np
# make some fake data
t = np.sort(np.random.rand(100))
y = np.cos(2 * np.pi * 10 * t)
y += np.random.randn(len(t))
dy = np.ones_like(t)
# start a conditional entropy process
proc = ConditionalEntropyAsyncProcess(phase_bins=10, mag_bins=5)
# format your data as a list of lightcurves (t, y, dy)
data = [(t, y, dy)]
# run the CE process with your data
results = proc.run(data)
# finish the process (probably not necessary but ensures
# all data has been transferred)
proc.finish()
# Results is a list of [(freqs, CE), ...] for each lightcurve
# in ``data``.
freqs, ce_spectrum = results[0]
If you want to run CE on large datasets, you can do
proc.large_run(data, max_memory=1e9)
instead of run
, which will ensure that the memory limit (1 GB in this case) is not exceeded on the GPU (unless of course you have other processes running).
[G2013] | Graham et al. 2013 |